It was a gorgeous, sunny late summer afternoon. Gin tonic in hand, I was chatting with two other wedding guests. We were an engineer and two scientists camouflaged under a nice suit and two summer dresses. And it happened again. The bubbles, the happy occasion and the sun were not enough to keep us away from a serious discussion. About data - and the future of it.
Data is not only problematic when it is owned by Facebook. The amount of data we produce is growing so much that storage is a concern. Remember: data centres also make tape copies of what is stored in their servers. And producing data is also costly. While the molecular storage field is trying to use DNA or fluorophores as more efficient ways to store data, most scientists are still stimulating each other to produce as much data as they can. After smoking, alcohol and red meat… is data our new addiction? Maybe we should talk about it in lab meetings, instead of at cocktail parties.
Let’s first take a step back. Are you sure that Meten is weten? I would like you to consider the practical link between data and knowledge. Imagine I give you a bunch of datapoints without telling you where they come from. The only knowledge you gain is not to trust me. Which might be useful for you, but… you know. Any resources that went into the production and storage of these data could be used in a much better way. That is not very sustainable.
Sadly, this happens frequently in research. Sample sizes we can’t draw any conclusions from problems finding or reproducing what the previous student did, results that will never see the light because they’re negative. Results stored in an obscure hard-drive somewhere, or behind a paywall. If data is supposed to become knowledge, it needs to be seen. Interpreted, combined, used. While we try to reduce plastic, fly less and eat less meat, we neglect the importance of thorough consideration when it comes to data production, storage, sharing and reproducibility.
My own examples make me feel uncomfortable, but I’ll share them. If we all put our uncomfortable results out there, others could focus on doing something better, right? To start with, I thought the FAIR criteria were not relevant for me. Three years into my PhD, I bumped into a database about cell-penetrating peptides. Ergo: there are more ways than you think to make your data Findable and Reusable. Maybe the FAIRshake tool offers some more.
Secondly, I spent four years of my PhD refusing to learn (the basics of) R. Before that, anything I’d done to my raw data was useless without access to GraphPad. In the end, a lockdown and the perseverance of a good friend made me become an R markdown fan. If we all used as much open software as possible, we would help the Interoperability principle. Bye-bye stress about the renewal of the institutional GraphPad license.
Third, I thought power calculations were just a requirement for animal study protocols. Actually, they can trigger us to think differently about experimental planning. So, which effects and how much variation do you expect, and what will really be biologically relevant? Needless to say: the perverse association we made between statistically significant and relevant is quite twisted. Constraints like costs and number of patients available are unavoidable issues. Then we should acknowledge them. We should be seeing pilots as a way to know the SD for the next power calculation. Instead, we are tacitly tweaking power calculations to get to the desired sample size.
Constraints should also be seen as a stimulus to sharing and reusing data. Here’s a publication showing new things by looking at already existing data. Also, results from control samples could be reused. But for that, you need to make sure that new and old data can be combined. Which brings me back to the wedding conversation. Is it so, as our engineer put it, that we should collect all the data we can, even if we’re not sure what we’ll use it for?
The idea behind this affirmation is that questions will arise, and otherwise AI will find hidden patterns that we can’t conceive. To me, this sounds again like an unsustainable addiction. Remember where I started, with that mysterious bunch of datapoints. Now imagine we invoke some AI power which can even infer what sort of data this is. Was it still worth it to create these data a priori and store them for a few years?
Seeing how fast techniques evolve, probably we can produce the same data better, faster and cheaper in the future. If we produce data when we need it, we save storage costs. However, for rare diseases it could be great to accumulate data: we’re all mortal, and patients do not come back. But can samples from 10 years ago be combined with new ones? Our enthusiastic engineer said that AI would help with this as well. To me, one thing is clear: without good data management, combining past and future datasets won’t work. Which ties back to the FAIR principles.
Also, the questions of the future may be related to phenomena that are not here yet. I’d rather make the choices I can make now. What can YOU do to make the most out of your scientific efforts? In the meantime, please save server pipette tips and server space for the next crisis. Drink less data but of better quality. Just like with good wine: cherish every bit and share great research with the world.
The Green Lab Initiative is a new collaboration of green enthusiasts at the Radboudumc who want to encourage people to go greener in the lab. With our green intentions of 2021, we try to inspire you every month to take small steps. Find us on Twitter or LinkedIn!
Blog by Estel Collado Camps
Related news items
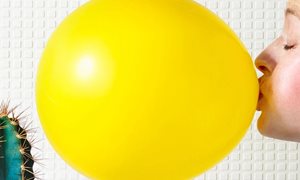
'If things grow wrong - The paradox of science' Estel Collado Camps visits a new exhibition at Museum De Lakenhal
9 December 2021 "In museum De Lakenhal, the exhibition If things grow wrong has opened its doors. One of the four installations catches our eye immediately: they call it The paradox of science. An evoking title, if you ask me… because the way we do, see and understand science knows many paradoxes." read more
Green Intentions December: ‘Tis the Season to Be Conscious Givers by Özlem Bulut
2 December 2021 The jolly holiday season is approaching, and our minds are busy with all kinds of gift ideas. Why not try to be more conscious givers this year and onward? In the last Green Intentions of the year, you’ll find sustainable gift ideas for your family, friends, and colleagues. read more
Green Intentions October - Sustainable office habits by Ilona Kessels
7 October 2021 In our monthly green blogs, we have been sharing a lot of sustainability tips for laboratories. But research is not only done in a laboratory. This time, we want to provide you some tips for a more sustainable office life. read more
Thoughtful Travel Pledge
8 September 2021 Good science comes with international collaborations and meetings. However, the carbon footprint of academic travel dwarfs the carbon footprint of entire countries. If we take the climate crisis seriously, this has to change. And it can change. How? Watch the clip! read more
Green Intentions September - Shut That Sash! by Özlem Bulut
2 September 2021 After a brief summer hiatus, we are back with our monthly green intentions. This time, we focus on the monster energy consumers of the lab life: fume hoods. read more