25 June 2020
Artificial intelligence (AI) is an important innovation in diagnostics, because it can quickly learn to recognize abnormalities that a doctor would also label as a disease. But the way that these systems work is often opaque, and doctors do have a better "overall picture" when they make the diagnosis. In a new publication, researchers from Radboudumc show how they can make the AI show how it’s working, as well as let it diagnose more like a doctor, thus making AI-systems more relevant to clinical practice.
[https://doi.org/10.1109/TMI.2020.2994463]
The two major differences compared to a human doctor are, first, that AI is often not transparent in how it’s analyzing the images, and, second, that these systems are quite "lazy". AI looks at what is needed for a particular diagnosis, and then stops. This means that a scan does not always identify all abnormalities, even if the diagnosis is correct. A doctor, especially when considering the treatment plan, looks at the big picture: what do I see? Which anomalies should be removed or treated during surgery?
How did the system learn to look at the same eye scan with ‘fresh eyes’? The system ignored the familiar parts by digitally filling in the abnormalities already found using healthy tissue from around the abnormality. The results of all the assessment rounds are then added together and that produces the final diagnosis. In the study, this approach improved the sensitivity of the detection of diabetic retinopathy and age-related macular degeneration by 11.2+/-2.0% per image. What this project proves is that it’s possible to have an AI system assess images more like a doctor, as well as make transparent how it’s doing it. This might help these systems become easier to trust and thus to be adopted by radiologists.
Background: what is 'deep learning'?
Deep learning is a term used for systems that learn in a way that is similar to how our brain works. It consists of networks of electronic ‘neurons’, each of which learns to recognize one aspect of the desired image. It then follows the principles of ‘learning by doing’, and ‘practice makes perfect’. The system is fed more and more images that include relevant information saying – in this case – whether there is an anomaly in the retina, and if so, which disease it is. The system then learns to recognize which characteristics belong to those diseases, and the more pictures it sees, the better it can recognize those characteristics in undiagnosed images. We do something similar with small children: we repeatedly hold up an object, say an apple, in front of them and say that it is an apple. After some time, you don't have to say it anymore – even though each apple is slightly different. Another major advantage of these systems is that they complete their training much faster than humans and can work 24 hours a day.
Photo caption: This series shows the four different stages of the same eye scan described in this article. From left to right: the original image; with highlights of anomalies by human experts; with highlights of anomalies by one scan by an AI system; with highlights of anomalies made by an iterative series of scans by an AI system. Note that the iterative process yields a more complete overview of the anomalies present than the single assessment.
Artificial intelligence (AI) is an important innovation in diagnostics, because it can quickly learn to recognize abnormalities that a doctor would also label as a disease. But the way that these systems work is often opaque, and doctors do have a better "overall picture" when they make the diagnosis. In a new publication, researchers from Radboudumc show how they can make the AI show how it’s working, as well as let it diagnose more like a doctor, thus making AI-systems more relevant to clinical practice.
[https://doi.org/10.1109/TMI.2020.2994463]
Doctor vs AI
In recent years, artificial intelligence has been on the rise in the diagnosis of medical imaging. A doctor can look at an X-ray or biopsy to identify abnormalities, but this can increasingly also be done by an AI system by means of "deep learning" (see ’Background: what is deep learning’ below). Such a system learns to arrive at a diagnosis on its own, and in some cases it does this just as well or better than experienced doctors.The two major differences compared to a human doctor are, first, that AI is often not transparent in how it’s analyzing the images, and, second, that these systems are quite "lazy". AI looks at what is needed for a particular diagnosis, and then stops. This means that a scan does not always identify all abnormalities, even if the diagnosis is correct. A doctor, especially when considering the treatment plan, looks at the big picture: what do I see? Which anomalies should be removed or treated during surgery?
AI more like the doctor
To make AI systems more attractive for the clinical practice, Cristina González Gonzalo, PhD candidate at the A-eye Research and Diagnostic Image Analysis Group of Radboudumc, developed a two-sided innovation for diagnostic AI. She did this based on eye scans, in which abnormalities of the retina occurred – specifically diabetic retinopathy and age-related macular degeneration. These abnormalities can be easily recognized by both a doctor and AI. But they are also abnormalities that often occur in groups. A classic AI would diagnose one or a few spots and stop the analysis. In the process developed by González Gonzalo however, the AI goes through the picture over and over again, learning to ignore the places it has already passed, thus discovering new ones. Moreover, the AI also shows which areas of the eye scan it deemed suspicious, therefore making the diagnostic process transparent.An iterative process
A basic AI could come up with a diagnosis based on one assessment of the eye scan, and thanks to the first contribution by González Gonzalo, it can show how it arrived at that diagnosis. This visual explanation shows that the system is indeed lazy – stopping the analysis after it as obtained just enough information to make a diagnosis. That’s why she also made the process iterative in an innovative way, forcing the AI to look harder and create more of a ‘complete picture’ that radiologists would have.How did the system learn to look at the same eye scan with ‘fresh eyes’? The system ignored the familiar parts by digitally filling in the abnormalities already found using healthy tissue from around the abnormality. The results of all the assessment rounds are then added together and that produces the final diagnosis. In the study, this approach improved the sensitivity of the detection of diabetic retinopathy and age-related macular degeneration by 11.2+/-2.0% per image. What this project proves is that it’s possible to have an AI system assess images more like a doctor, as well as make transparent how it’s doing it. This might help these systems become easier to trust and thus to be adopted by radiologists.
Background: what is 'deep learning'?
Deep learning is a term used for systems that learn in a way that is similar to how our brain works. It consists of networks of electronic ‘neurons’, each of which learns to recognize one aspect of the desired image. It then follows the principles of ‘learning by doing’, and ‘practice makes perfect’. The system is fed more and more images that include relevant information saying – in this case – whether there is an anomaly in the retina, and if so, which disease it is. The system then learns to recognize which characteristics belong to those diseases, and the more pictures it sees, the better it can recognize those characteristics in undiagnosed images. We do something similar with small children: we repeatedly hold up an object, say an apple, in front of them and say that it is an apple. After some time, you don't have to say it anymore – even though each apple is slightly different. Another major advantage of these systems is that they complete their training much faster than humans and can work 24 hours a day.
Photo caption: This series shows the four different stages of the same eye scan described in this article. From left to right: the original image; with highlights of anomalies by human experts; with highlights of anomalies by one scan by an AI system; with highlights of anomalies made by an iterative series of scans by an AI system. Note that the iterative process yields a more complete overview of the anomalies present than the single assessment.
Related news items

Surprisingly dominant cause underlying type I congenital defect of glycosylation
21 October 2021 Alex Garanto, Melissa Bärenfänger, Mirian Janssen, and Dirk Lefeber published a new study, identifying a surprisingly dominant genetic cause underlying type I congenital defect of glycosylation with neuromusculoskeletal phenotypes. read more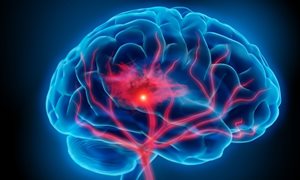
Treatment of brain diseases at risk if neuroscientists can no longer conduct their research
5 August 2021 In order to treat brain diseases such as depression, Alzheimer's or ADHD, more research into the brain is needed. read more
Retina-on-chip modeling and treating eye diseases in a dish
10 June 2021Alex Garanto, together with Anneke den Hollander and Andries van der Meer (UTwente) have received 1,17M€ to develop a retina-on-chip model to improve our understanding in eye diseases and to develop treatments against them.
read more
Innovative therapy developed for the future treatment of hereditary (deaf)blindness
26 May 2021 Researchers from the department of Otorhinolaryngology, headed by Erwin van Wijk, developed a novel therapeutic strategy to halt the progression or to prevent vision loss in patients as a consequence of USH2A exon 13 mutations. read more
Foundation Fighting Blindness Awards 1.4 million dollars for Rob Collin and Carel Hoyng
29 April 2021 Two researchers from the Radboudumc have received a prestigious award from the Translational Research Acceleration Program of the Foundation Fighting Blindness USA. read more